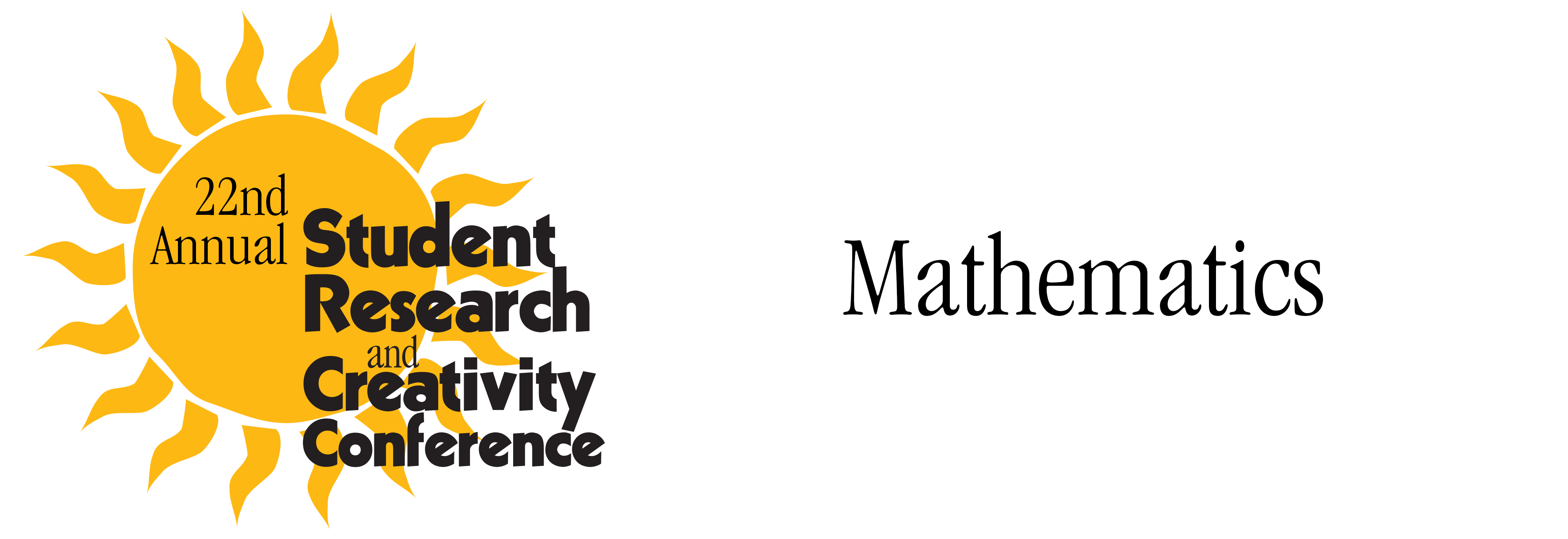
-
Analysis of Transportation Rates of New Markets
Xianglin Kong
Xianglin Kong, Applied Mathematics
Faculty Mentor: Professor Joaquin Carbonara, Mathematics
Transportation rates are involved in the management of almost all large enterprises, and they are an important part of cost considerations. Calculating and forecasting transportation rates can play an important role in the future planning of an enterprise. The development of enterprises has put forward higher requirements for logistics, distribution and cargo storage, which requires the establishment of more advanced and scientific distribution center solutions. In this project, we use Python, the historical data of the enterprise, and established various models to calculate the transport rate and accurately depict transportation rates in new regions. My project focuses on less than truckload (LTL) transportation mode and analyzes the relationship between each factor to draw formulas. The parameters of the models were determined and compared. Then the model with the best fits was found and corresponding predictions were made. -
The Models for the Average Number of Applicants at Ivy League Schools
Gretchen Mann
Gretchen Mann, Applied Mathematics
Faculty Mentor: Professor Bruce Swan, Mathematics
Within the past couple of decades, attending any one of the eight Ivy League schools has been increasingly more competitive and the admission rates have been declining year after year. This is primarily due to more prospective students applying than in previous decades. While the average number of students that an Ivy League school accepts in any given year is approximately consistent, the average number of applications received has been trending positively. As a result, the average admission rates for Ivy League schools have been gradually declining since 2003. By using SPSS and creating a multivariate time-series regression model, my project forecasts the average number of Ivy League school applicants for the 2020-2021 academic year. Other potential time-dependent variables will be introduced too, such as the total number of high school graduates, SAT and ACT scores, average grade point average (GPA), mean parental income, average financial aid package awarded, and average indebtedness after graduation. Potential models were created based on these predictors, using stepwise regression methods such as forward selection and backward elimination. The ultimate goal of this paper is aimed towards choosing and analyzing a best-fit model, based on the criteria of how significant it is at predicting the average number of applicants and how the optimal model fits compared to other potential models. Multicollinearity between the regressor variables was also analyzed in the models. -
Finding Optimal Transportation Rates in New Regions
Lewen Yin
Lewen Yin, Applied Mathematics
Faculty Mentor: Professor Joaquin Carbonara, Mathematics
The purpose of my project was to predict transportation rates in new regions for existing business models. In the current market, many supply-chain models exist to support managerial decisions - expansion, closure, acquisition, and organic growth. In most cases, the current state is not optimal in terms of cost, efficiency, or service. Network modeling enables us to diagnose the current state and examine what alternative scenarios might look like. Currently, Rich’s has transportation rates for existing markets. They need to expand their major Distribution Centers (DCs). They have historical transportation rates into those markets for the weight brackets and transportation modes (Truckload TL, Multi-Stop TL, Less than Truckload LTL, Intermodal IM) that they use. When they do network design problems and try to assess if there should be a new DC added, they have historical transportation rates to use for the potential lanes. So, we need to use data analysis methods and the historical rates to predict costs for new lanes. Rather than using the straight market rates, we run a comparison between historical rates and the market rates from TransPlace for the same lanes, to get a ratio, such as 1.1 or 0.87, depending on mode. In this project, we focus on the MSTL mode (Multi Stop Truck Loads), and we use Python to process data analysis and prediction. Then we discuss how to create a better approach for calculating the ratio for the optimization of urban transportation. We first need to create a better approach for calculating the ratio, then we need to explore differences in high density lanes (frequency of shipments), variations in load size, and market regions (urban to rural and vice versa). Finally, we incorporate external data (population density, employment rate, etc.) into the prediction. -
Predicting Rich’s Transportation Rates of Unknown Markets
Boya Zhang
Boya Zhang, Applied Mathematics
Faculty Mentor: Professor Joaquin Carbonara, Mathematics
Rich Products Corporation (also known as Rich's) is a privately held, multinational food-products corporation headquartered in Buffalo, New York. The problem of predicting transportation rates is receiving considerable attention with the establishment of new markets in the United States and Canada. Expanding new markets requires the creation of a new distribution center (DC), and its associated cost of transportation to the point of delivery. The goals of this project were to: (1) analyze historical transportation rates in the south-east and south-central regions; (2) determine appropriate weight brackets and transportation modes (Truckload: TL; Multi-Stop Truckload: MSTL; Less than Truckload: LTL; Intermodal: IM); and (3) use Market rates from the TransPlace database to predict transportation rates for new markets. The current project focuses on TL transportation.